A view on predictive maintenance
Predictive Maintenance
At HVR Engineering we experience a change in the way of working between the machine suppliers and their customers. A development in this relation is that customers demand for more control over the operational functionality of their machines, instead of leaving this in the hands of the supplier.
In the past it was often accepted that machines had unannounced downtime once in a while. Nowadays machines become more and more complex, with more interaction between components and tighter machine-to-machine interaction. This means that a failure of whatever small component can already have a major impact on the throughput of a manufacturing plant or it can cause serious project delays.
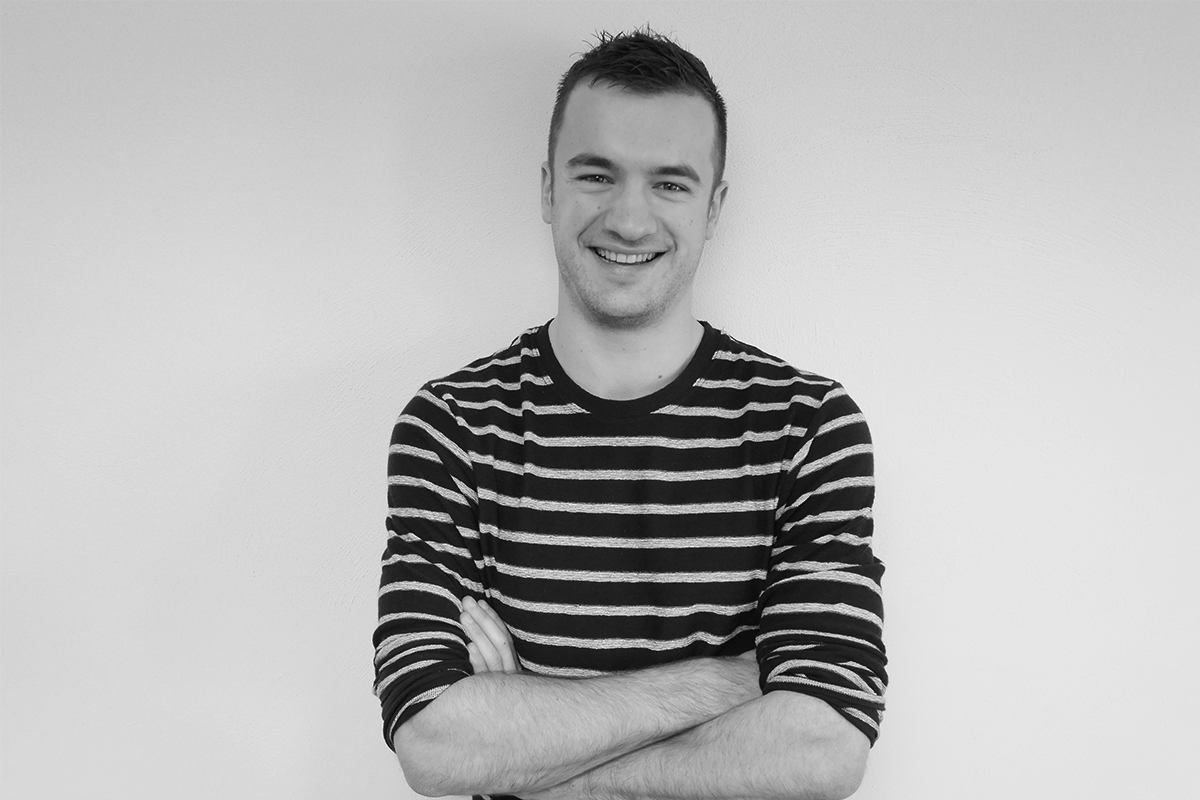
Jeroen Ligthart
Lead Engineer Simulation
"In contrast to preventive maintenance, predictive maintenance relies on machine operation data and measurement data to evaluate the machine performance in real-time."
Preventive maintenance
A rather simple approach to reduce the downtime of a machine is to perform preventive maintenance: By balancing the cost of replacement of components with the cost of a downtime, a maintenance schedule can be created that defines for all components at what interval they need replacement.
Preventive maintenance has some notable disadvantages though. The cost to replace a single component may be rather limited, but in total a preventive maintenance schedule can result in quite significant maintenance expenses. And even then a preventive maintenance schedule does not guarantee to avoid downtime: New components have high initial failure rates due to infant-mortality failures (according to the bathtub curve model) which are not accounted for by a preventive maintenance strategy. Also random failures can occur at any instance, so also before the preventive maintenance schedule proposes to replace the part.
Predictive maintenance
A technically more advanced method to meet the customer’s downtime expectations can be a predictive maintenance approach. In contrast to preventive maintenance, predictive maintenance relies on machine operation data and measurement data to evaluate the machine performance in real-time. If the data is analyzed successfully, even infant-mortality failures can be avoided. Furthermore, well-functioning components do not need to be replaced at a fixed interval which saves costs and reduces the number of required maintenance shut-downs.
A predictive maintenance strategy can be set up in a variety of ways. Some examples are given below.
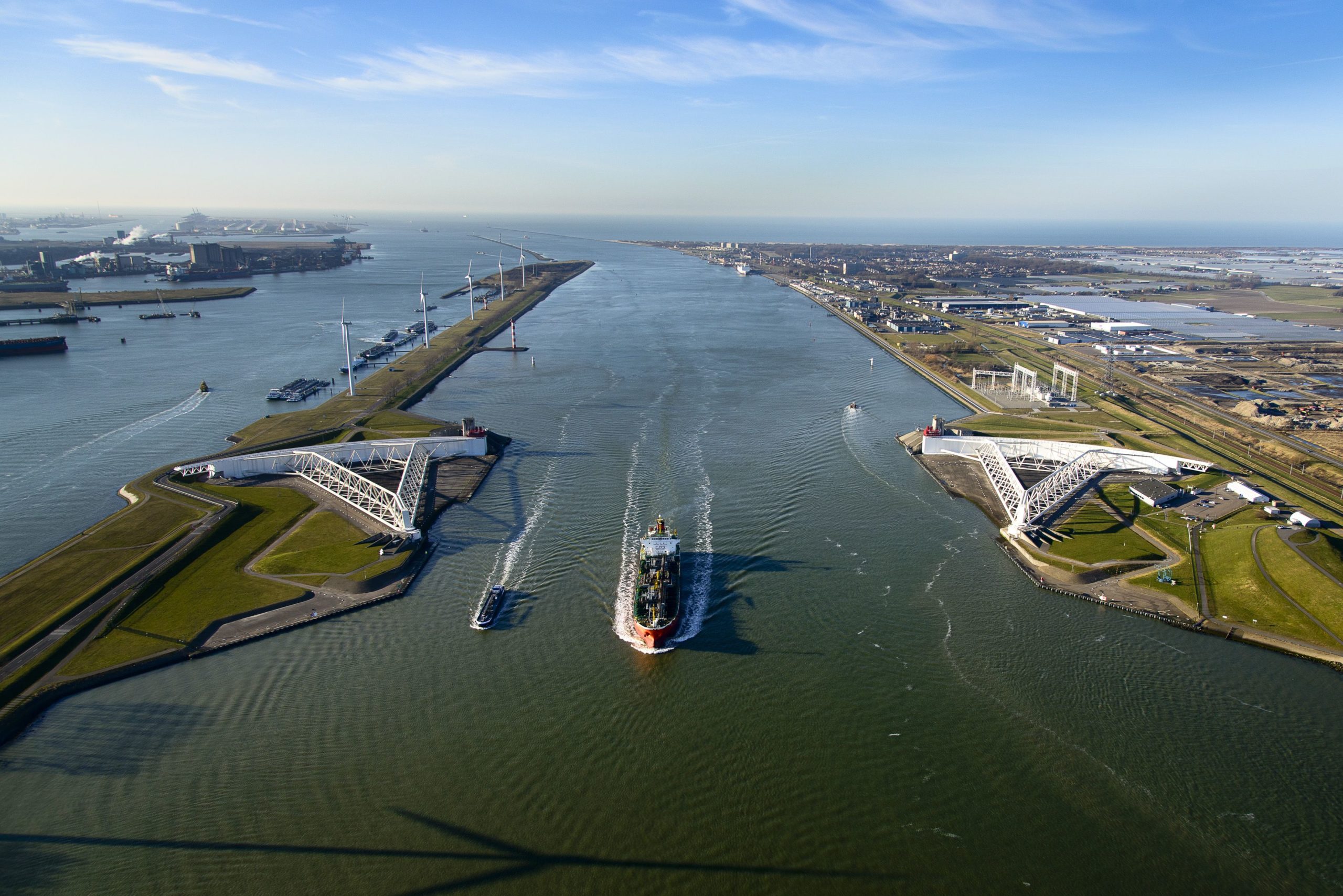
Component-based predictive maintenance
A relativity simple predictive maintenance approach is to perform measurements on individual components, often the rotating parts of a machine, like pumps, fans, and gear boxes. The applied measurement type is typically acceleration (or vibration), temperature, or acoustic. Trends are established in the collected data to map the condition of the component. The trends provide maintenance alerts or, as a hybrid solution, is used to optimize the system’s maintenance interval. This approach may be called Condition-Based Monitoring as well.
Formula-1 fans might recognize this way of working: The drivers are called to the pit lane for a new set of tires if the car’s vibrations levels increase over a certain threshold. Verstappen fans will know as well that not all failures announce themselves in this way; In the Azerbaijan Grand Prix Max’s tire blew while the team did not report any changes in the vibration levels. A proper predictive maintenance approach reduces these random unannounced failures to a minimum.
Advanced predictive maintenance
The conventional component-based predicative maintenance strategy has some limitations:
- Not always can a machine be equipped with a large number of sensors on all relevant moving parts. As discussed in this other blog, in a harsh environment, sensors break down easily. Malfunctioning sensor trigger unnecessary (and costly) maintenance actions in a predictive-maintenance system. Also it can be (too) expensive to equip a machine with a large number of sensors.
- Another problem with a conventional predictive maintenance strategy is that if a machine is subjected to large variations in its operation point, trending vibration levels will by itself not lead to proper information on the component functionality. In this case the operation performance cannot be analyzed for individual components, but only for the machine as a whole.
- Finally, conventional component-based predicative maintenance relies on a large dataset, so it requires long-term and continuous machine operation. With a small dataset conventional techniques will not be able to find trends in the data.
These type of systems require a more advanced predictive maintenance technique. This is where HVR can add value, using the years of experience with power transmission systems. Two examples of HVR’s solutions for advanced predictive maintenance strategies are as follows:
- If a machine cannot be equipped with a large number of sensors, a model-based predictive maintenance approach can be realized: If a limited real-time data set is fed through a digital twin simulation model, the simulation generates virtual measurement data of the machines internal components (that are themselves not instrumented). So even components that are not accessible for sensor instrumentation can be performance-monitored. This approach requires extensive system knowledge, extensive knowledge on performing measurements, and above all experience with power transmission systems. With this knowledge in house, HVR Engineering tackles this problem for IQIP’s Hydrohammer.
- Another advanced predictive maintenance solution where HVR Engineering is involved considers the Maeslantkering storm surge barrier. The difficulty with this project is that storm surge barriers are operated at an extremely large time interval, so measurement data is sparse. Simple trend-finding predictive maintenance algorithms are therefore not a solution. Instead, HVR has set up data analyses methods which are based on extensive mechanical- and hydraulic systems knowledge. These algorithms manages to monitor the condition of the system components, despite having such a small dataset.
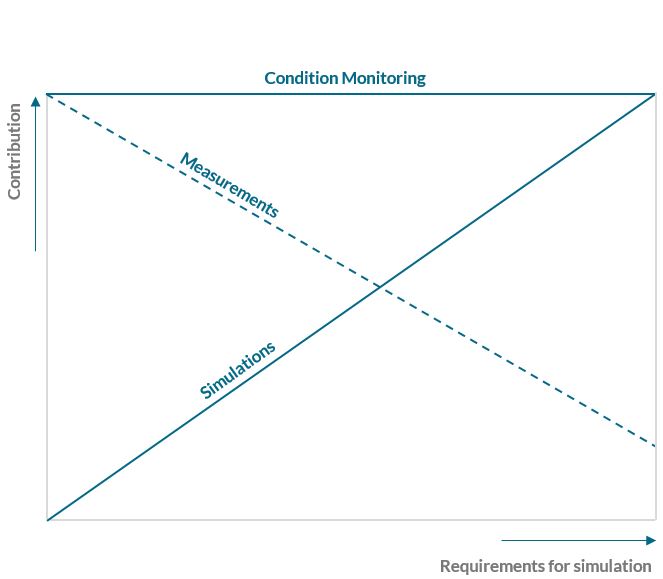
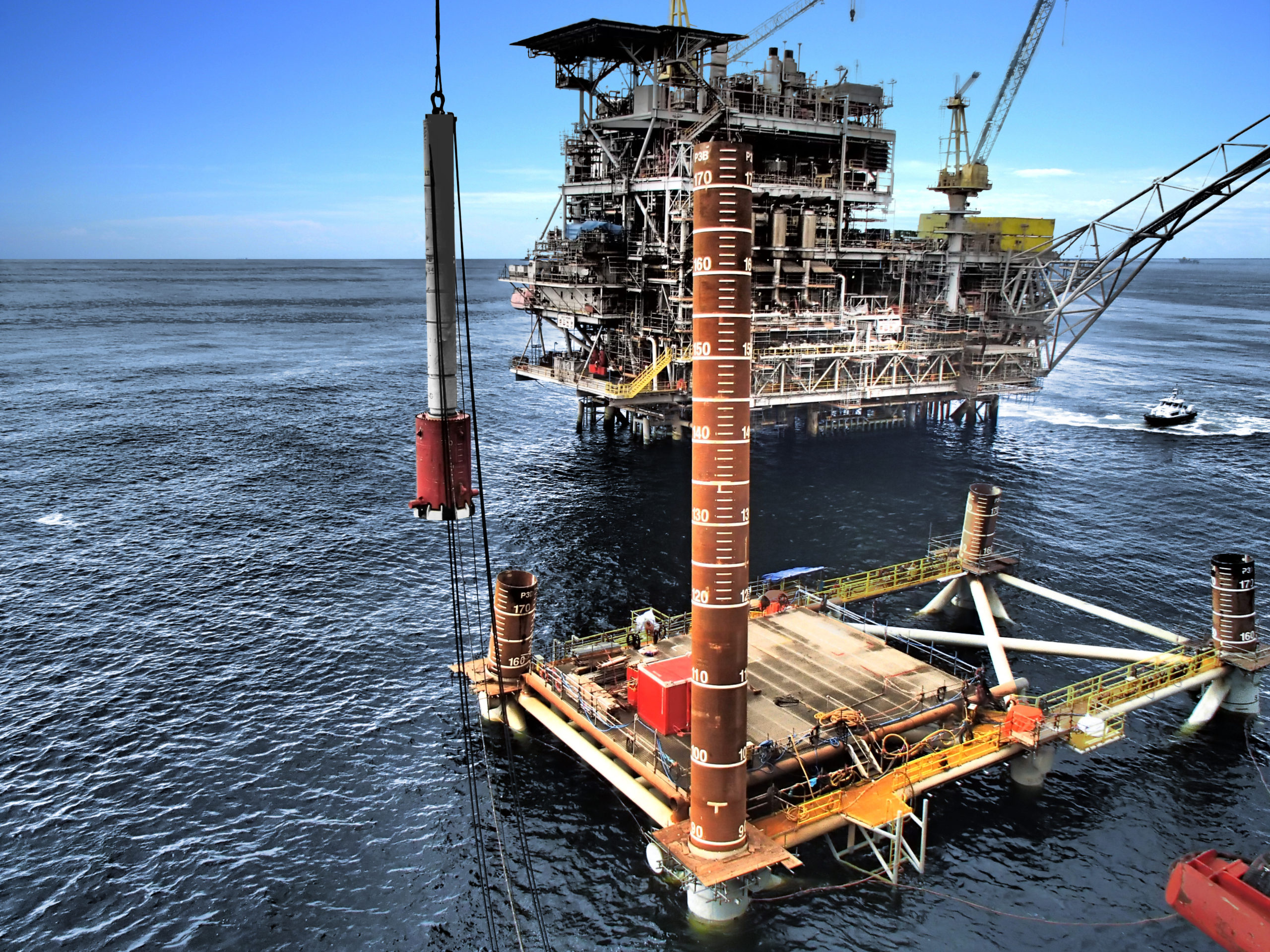
Simulations
Our simulation models can support with advanced predictive maintenance when data is not available. Read more about this:
How we can help you
At HVR Engineering we believe that understanding a process is essential to deliver accurate and realistic results. Our experience with interpretation of the dynamics in a system, either via data analysis or simulation model development gives the best solutions for setting up your predictive maintenance programs. Interested? Get in touch!